Tired of your data gathering dust ?
Lets put it to work with AI
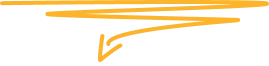
Talk to our Enterprise GPT Specialists!
Discover why agentic AI implementations often fail, uncovering the hidden prerequisites essential for building adaptive, dynamic systems that prioritize relevance over data volume.
Why is AI important in the banking sector? | The shift from traditional in-person banking to online and mobile platforms has increased customer demand for instant, personalized service. |
AI Virtual Assistants in Focus: | Banks are investing in AI-driven virtual assistants to create hyper-personalised, real-time solutions that improve customer experiences. |
What is the top challenge of using AI in banking? | Inefficiencies like higher Average Handling Time (AHT), lack of real-time data, and limited personalization hinder existing customer service strategies. |
Limits of Traditional Automation: | Automated systems need more nuanced queries, making them less effective for high-value customers with complex needs. |
What are the benefits of AI chatbots in Banking? | AI virtual assistants enhance efficiency, reduce operational costs, and empower CSRs by handling repetitive tasks and offering personalized interactions. |
Future Outlook of AI-enabled Virtual Assistants: | AI will transform the role of CSRs into more strategic, relationship-focused positions while continuing to elevate the customer experience in banking. |
"Just dump more data into it."
These five words have derailed more agentic AI implementations than any technical failure. Fortune 500 companies pour millions into sophisticated data infrastructure, only to watch their agentic systems falter against seemingly simpler solutions. The culprit isn't technical capability—it's a fundamental misunderstanding of how agentic systems actually build and maintain intelligence.
Traditional enterprise AI systems operate like libraries – static, organized, and predictable. Agentic AI demands something entirely different: a living, breathing knowledge ecosystem that's more akin to a newsroom during breaking news. When breaking news hits, no one cares about the archived stories – they care about what's happening right now, how it connects to recent events, and what it might mean for tomorrow.
A global financial institution demonstrated this reality starkly during the COVID-19 market volatility (Financial Times, 2021). Their sophisticated AI trading systems, despite massive data infrastructure, required fundamental recalibration when market patterns shifted dramatically. The solution wasn't more data—it was changing how their systems processed and prioritized information in real time.
This fundamental misalignment between data volume and intelligence manifests in three critical ways:
Most current architectures actively fight against real-time knowledge integration. While they excel at storing and retrieving information, they struggle with microsecond pattern recognition and dynamic context switching.
The result? Systems that know everything about yesterday but nothing about today.
Here's a stark reality: Most validation frameworks are built for yesterday's truth. In agentic systems, truth has an expiration date – and it's getting shorter by the day. This isn't just about data freshness; it's about the fundamental nature of truth in dynamic business environments.
Microsoft's 2024 Enterprise AI Implementation Report revealed a critical insight: systems maintaining static knowledge bases showed 47% accuracy degradation over just three months, regardless of their initial performance levels. The problem wasn't degrading data—it was static learning infrastructure.
Modern agentic systems must challenge their assumptions hourly, tracking truth decay rates across contexts and maintaining multiple concurrent truth states. This isn't just good practice—it's survival.
Modern agentic systems must master a skill that most AI platforms actively resist: strategic forgetting. When we analyze successful implementations, a counterintuitive pattern emerges systems that forget strategically consistently outperform those that simply accumulate knowledge.
This means:
Strip away the marketing speak, and here's what most "continuous learning" systems actually do: they ingest new data indiscriminately, add it to existing patterns without questioning relevance, and adjust weights marginally while maintaining outdated assumptions. That's not learning – that's hoarding with extra steps.
Before proceeding further, assess your organization's readiness through these critical questions:
Your answers here reveal your system's fundamental ability to adapt to business change. Most organizations discover their systems excel at stability but fail at evolution.
These questions expose the gap between technical accuracy and business relevance—a gap that widens with every market shift and business evolution
While security teams obsess over external threats, the biggest risk to agentic systems lurks within: the speed of business evolution. Every strategic pivot, market adjustment, and process refinement creates ripple effects that most systems aren't designed to handle.
The path to effective implementation isn't about building perfect systems—it's about building systems that perfect themselves. Success requires three core characteristics that today's architectures actively resist:
Your system must know what it doesn't know. This means:
Adobe's enterprise AI systems demonstrated this principle in action. According to their 2023 Technical Brief, implementing automated limitation detection reduced critical AI errors by 64%. The key wasn't better algorithms—it was better self-awareness.
The distinction between traditional AI stability and agentic evolution becomes most apparent under stress. Performance under pressure isn't optional. Your system must:
When business conditions change rapidly, stable systems maintain their performance metrics while delivering increasingly irrelevant results. Evolution-capable systems might show temporary metric fluctuations but maintain business relevance.
This evolution capability requires a fundamental shift in how we think about system architecture:
- From static validation to dynamic truth assessment
- From performance stability to adaptive relevance
- From data accumulation to strategic knowledge management
Think of it as the difference between a static map and a real-time navigation system. The map might be perfectly accurate but becomes increasingly less useful as roads change. The navigation system, while sometimes less certain, maintains its practical value by constantly updating its understanding.
Implementation follows three distinct phases, and each phase presents unique challenges.
During the Foundation Phase, organizations often struggle with the apparent paradox of building for change rather than stability.
Success requires: - Establishing flexible knowledge frameworks - Creating adaptive validation mechanisms - Implementing responsive learning protocols.
Without this foundation, other improvements become temporary patches rather than permanent solutions.
The Integration Phase demands a delicate balance between maintaining current operations and enabling evolution.
Organizations must: - Align business metrics with evolution capabilities - Create safe spaces for system adaptation - Build bridges between technical and business outcomes
The Evolution Phase is where most implementations either thrive or fail. Success depends on: - Continuous alignment with business objectives - Active monitoring of evolution effectiveness - Regular calibration of learning mechanisms
Three immediate actions separate organizations that succeed with agentic AI from those that fail:
The competitive advantage doesn't lie in data volume or algorithmic complexity—it lies in building systems that embrace continuous evolution, prioritize knowledge velocity over volume, and implement strategic forgetting protocols.
Want to understand where your agentic AI system stands? Our team can conduct a rapid capability assessment that reveals your system's evolution readiness and provides a clear roadmap for implementation success.
The future belongs to systems that evolve. Is yours ready?
Fluid AI is an AI company based in Mumbai. We help organizations kickstart their AI journey. If you’re seeking a solution for your organization to enhance customer support, boost employee productivity and make the most of your organization’s data, look no further.
Take the first step on this exciting journey by booking a Free Discovery Call with us today and let us help you make your organization future-ready and unlock the full potential of AI for your organization.
AI-powered WhatsApp community for insights, support, and real-time collaboration.
Talk to our Enterprise GPT Specialists!
AI-powered WhatsApp community for insights, support, and real-time collaboration.