Tired of your data gathering dust ?
Lets put it to work with AI
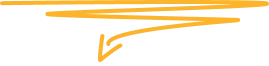
Talk to our Enterprise GPT Specialists!
Understanding how Gen AI systems arrive at their decisions is crucial for building trust in their outcomes. XAI helps shed light on the "why" behind an AI decision,
Why is AI important in the banking sector? | The shift from traditional in-person banking to online and mobile platforms has increased customer demand for instant, personalized service. |
AI Virtual Assistants in Focus: | Banks are investing in AI-driven virtual assistants to create hyper-personalised, real-time solutions that improve customer experiences. |
What is the top challenge of using AI in banking? | Inefficiencies like higher Average Handling Time (AHT), lack of real-time data, and limited personalization hinder existing customer service strategies. |
Limits of Traditional Automation: | Automated systems need more nuanced queries, making them less effective for high-value customers with complex needs. |
What are the benefits of AI chatbots in Banking? | AI virtual assistants enhance efficiency, reduce operational costs, and empower CSRs by handling repetitive tasks and offering personalized interactions. |
Future Outlook of AI-enabled Virtual Assistants: | AI will transform the role of CSRs into more strategic, relationship-focused positions while continuing to elevate the customer experience in banking. |
Understanding how these systems arrive at their decisions is crucial for building trust in their outcomes. XAI helps shed light on the "why" behind an AI decision, fostering transparency and allowing stakeholders to scrutinize and understand the reasoning process.
Businesses are increasingly using AI to bring automation, enhace their decision making & increase workflows speed & productivity of every employee. But how do AI derive at their conclusions? What data do they use? And can we trust the results?
The whole calculation process is turned into what is commonly referred to as a “black box" that is impossible to interpret. These black box models are created directly from the data. And, not even the engineers or data scientists who create the algorithm can understand or explain what exactly is happening inside them or how the AI algorithm arrived at a specific result.
Further, organizations that practices making AI explainable are more likely to see their annual revenue and EBIT grow at rates of 10 percent or more.
Seventy-three percent of US companies have already adopted AI in at least some areas of their business, according to 2023 Emerging Technology Survey — and Generative AI is leading the way. One year after ChatGPT hit the market, more than half of the companies we surveyed (54%) have implemented GenAI in some areas of their business. (PwC 2024 AI Business Predictions)
The increasing adoption of AI is accompanied by a growing emphasis on responsible development and deployment, including explainability (XAI) for GenAI models.
XAI aims to make the complex decision-making processes of artificial intelligence (AI) systems more understandable and transparent to humans. With XAI, human can comprehend & trust the output of AI technology
With XAI, we want to answer questions like:
Unlike discriminative AI models that classify or predict, GenAI models create new data (e.g., images, text, code). Their complex internal processes can be opaque, GenAI's "black-box" nature makes it difficult to understand how they arrive at specific outputs.
Understanding how these systems arrive at their decisions is crucial for building trust in their outcomes. XAI helps shed light on the "why" behind an AI decision, fostering transparency and allowing stakeholders to scrutinize and understand the reasoning process.
Developing models that not only generate outputs but also provide explanations alongside them. XAI helps bridge this gap by providing insights.
Explainable AI is one of the key requirements for implementing responsible AI, a methodology for the large-scale implementation of AI methods in real organizations with fairness, model explainability and accountability.
Challenges in Explaining Complex Outputs:
GenAI models often create subjective and creative outputs (e.g., artwork, music, creative text formats). Quantifying the reasoning behind such outputs can be challenging, making it difficult to provide clear and concise explanations using current XAI techniques.
Balancing Explainability and Creativity:
Enforcing high explainability standards might stifle the very creativity and novelty that GenAI models are known for. Striking the right balance between providing adequate explanations and preserving the model's creative potential could be challenging
Limited Interpretability of "Black-Box" Models:
Some advanced GenAI models, particularly deep learning models, can be highly complex with intricate internal workings. These "black-box" models may pose a challenge for XAI techniques, making it difficult to extract meaningful explanations for their outputs.
Subjectivity and User Bias:
The perceived value of explanations can be subjective and influenced by the training data. Additionally, users might unknowingly introduce bias into their interpretation of explanations, leading to misinterpretations or misplaced trust.
Interpretable and inclusive AI
Fluid AI has built interpretable and inclusive AI systems for Organisations with tools designed to help detect the resonability behind the output and provide user with the insight needed to trust the output
Deploy AI with confidence
To grow end-user trust, especially in critical decision-making domains, we have improved transparency with explanations of the llm models, get a prediction and a score in real time indicating which data affected the final result.
Enhancing GPT Outputs with Anti-Hallucination Insights
Large language models (LLMs), sometimes generate outputs that are creative but lack factual basis ("hallucination"). With Fluid AI’s XAI technique pinpoint which parts of the input data have the most significant influence on the model's output along with indicating where the model might be "hallucinating", this help model to produce more reliable and trustworthy outputs.
Fluid AI has built controls which can be enable to Reduce Hallucination and Derive Output on Factual Data
The increasing market size and growth projections for XAI solutions suggest growing adoption. MarketsandMarkets forecasts the Explainable AI market to reach $16.2 billion by 2028, indicating a rising demand for XAI technologies
By bridging the gap between the complex world of GenAI and human understanding, XAI holds immense potential to foster trust, collaboration, and responsible use of this powerful technology.
Overall, XAI acts as a bridge, empowering organizations to use GenAI/LLM models with confidence and trust by fostering transparency, enabling responsible development, and facilitating continuous improvement. This allows organizations to unlock the full potential of GenAI while mitigating risks and ensuring ethical use within the enterprise landscape.
At Fluid AI, we stand at the forefront of this AI revolution, making the Gen AI Explainable & Transparent for Enterprise-usecases. We help organizations kickstart their AI journey, if you’re seeking a solution for your organization, look no further. We’re committed to making your organization future-ready, just like we’ve done for many others.
Take the first step towards this exciting journey by booking a free demo call with us today. Let’s explore the possibilities together and unlock the full potential of AI for your organization. Remember, the future belongs to those who prepare for it today.
AI-powered WhatsApp community for insights, support, and real-time collaboration.
Talk to our Enterprise GPT Specialists!
AI-powered WhatsApp community for insights, support, and real-time collaboration.