Tired of your data gathering dust ?
Lets put it to work with AI
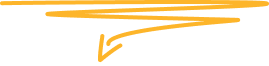
Talk to our Enterprise GPT Specialists!
Explore the evolution of agentic AI, its principles, dimensions, and future trajectory toward adaptive, purpose-driven, and collaborative intelligence systems.
Why is AI important in the banking sector? | The shift from traditional in-person banking to online and mobile platforms has increased customer demand for instant, personalized service. |
AI Virtual Assistants in Focus: | Banks are investing in AI-driven virtual assistants to create hyper-personalised, real-time solutions that improve customer experiences. |
What is the top challenge of using AI in banking? | Inefficiencies like higher Average Handling Time (AHT), lack of real-time data, and limited personalization hinder existing customer service strategies. |
Limits of Traditional Automation: | Automated systems need more nuanced queries, making them less effective for high-value customers with complex needs. |
What are the benefits of AI chatbots in Banking? | AI virtual assistants enhance efficiency, reduce operational costs, and empower CSRs by handling repetitive tasks and offering personalized interactions. |
Future Outlook of AI-enabled Virtual Assistants: | AI will transform the role of CSRs into more strategic, relationship-focused positions while continuing to elevate the customer experience in banking. |
The conversation around AI evolution has been dominated by discussions of larger models and expanded datasets. But true evolution in AI systems, particularly agentic ones, represents something fundamentally different. It's time to explore what real evolution means in the context of artificial intelligence.
Evolution in natural systems provides us with a powerful metaphor for understanding how AI systems should develop. While biological evolution occurs through random mutations and natural selection over generations, AI evolution operates on fundamentally different principles:
Unlike biological evolution's random mutations, agentic AI systems evolve with purpose and direction. Imagine a master craftsman who doesn't just randomly try new techniques, but deliberately develops specific skills to meet new challenges. These systems analyze performance gaps and business needs, then develop targeted capabilities to address them.
Take a manufacturing quality control system, for instance. When faced with new product variations, it doesn't just randomly adjust its parameters. Instead, it systematically identifies specific inspection requirements, develops precise detection capabilities, and validates these changes before full implementation. This directed approach ensures that every evolutionary step adds concrete value to the operation.
The validation process is particularly crucial. Before any new capability is fully integrated, it undergoes rigorous testing in a controlled environment. This means changes aren't just made—they're proven effective before deployment, significantly reducing the risk of performance degradation.
While natural evolution takes generations to produce meaningful changes, agentic AI systems evolve in real-time. This continuous evolution happens while the system maintains its existing capabilities—think of it as upgrading an airplane while it's still flying.
The true power of real-time development lies in its multi-dimensional nature. While the system might be improving its pattern recognition in one area, it could simultaneously be enhancing its decision-making capabilities in another. This parallel evolution allows for rapid adaptation to changing business needs without disrupting current operations.
The system constantly monitors its performance across various metrics, identifying opportunities for improvement and implementing changes on the fly. This real-time adaptation ensures that the system remains relevant and effective, even as business conditions change.
Evolution in agentic AI isn't just about technical improvements—it's about creating measurable business value. Each new capability is integrated into the system with clear purpose, ensuring it aligns with broader business objectives.
The integration process follows a systematic approach: First, new capabilities are evaluated against specific business metrics. Then, they're gradually integrated into the system's operations, with continuous monitoring to ensure they enhance rather than disrupt existing processes. Finally, the impact is measured not just in technical terms, but in concrete business outcomes.
Knowledge evolution in agentic AI goes far beyond simple data accumulation. The system actively processes and updates its understanding in real-time, similar to how a human expert continuously refines their knowledge through experience.
This dynamic understanding manifests in three key ways:
First, the system continuously updates its knowledge base with new information, ensuring its understanding remains current.
Second, it builds contextual relationships between different pieces of information, creating a rich web of understanding.
Finally, it automatically identifies and deprecates outdated information, preventing the accumulation of irrelevant knowledge.
Capability evolution represents the system's ability to develop and enhance its skills over time. This isn't just about getting better at existing tasks—it's about developing entirely new abilities in response to emerging needs.
The process is remarkably sophisticated. When the system encounters a new challenge, it doesn't just try to force-fit existing solutions. Instead, it analyzes the requirement, develops specific capabilities to address it, and then enhances these capabilities through experience. Moreover, successful approaches in one domain can be adapted and applied to others, creating a cross-pollination effect that accelerates overall system development.
Integration evolution ensures that as individual components of the system improve, their collective performance grows proportionally. This dimension focuses on how different parts of the system work together, creating a whole that's greater than the sum of its parts.
The system continuously optimizes how its components interact, improving coordination and resource utilization. This leads to enhanced handling of complex scenarios that require multiple capabilities working in concert. Think of it as an orchestra where each musician not only improves individually but also gets better at playing with others, creating increasingly sophisticated performances.
Through these three dimensions, agentic AI systems achieve a form of evolution that's both more directed and more rapid than anything possible in natural systems. This evolutionary capability ensures that these systems don't just maintain their relevance—they continuously enhance their value to the organizations they serve.
Horizontal scaling in evolving AI systems goes far beyond simply handling more data or users. It's about expanding the system's operational domain while maintaining effectiveness. This expansion happens organically, with the system gradually extending its capabilities into adjacent areas through a process of controlled exploration and validation.
Consider how a system might expand from basic document processing to handling complex regulatory compliance. It doesn't make this leap in one giant step—instead, it progressively builds understanding and capability, ensuring stability at each stage before moving forward.
Vertical scaling represents the deepening of expertise within specific domains. This process mirrors how human experts develop deep specialization, but at a greatly accelerated pace. The system doesn't just accumulate more data about a domain—it develops increasingly sophisticated models of how things work.
This deepening expertise manifests in several ways:
Perhaps the most powerful aspect of scaling in agentic AI systems is the network effect—how improvements in one area catalyze advancements in others. When multiple evolving systems interact, they create a multiplication effect where insights and capabilities spread rapidly across the network.
This collaborative evolution enables:
Implementing evolutionary AI systems requires a sophisticated approach to measuring progress. Traditional metrics like accuracy and processing speed, while still relevant, are no longer sufficient. We need to evaluate:
The system's ability to identify new patterns and opportunities. This isn't just about spotting trends—it's about understanding their significance and potential impact. Evolution assessment looks at both the speed and quality of pattern recognition, measuring how quickly and accurately the system can identify meaningful changes in its environment.
Adaptation effectiveness becomes a critical metric. How well does the system modify its behavior based on new information? More importantly, how does it balance the need to adapt with the requirement to maintain stable performance in critical areas?
Successful implementation of evolving AI systems requires a careful balance between ambition and practicality. Organizations need to:
First, establish clear evolutionary objectives that align with business goals. These objectives should be specific enough to guide development but flexible enough to accommodate unexpected opportunities for growth.
Second, create a supportive environment for evolution. This means building infrastructure that can handle changing requirements and establishing governance frameworks that encourage rather than restrict beneficial changes.
Finally, develop metrics that meaningfully capture evolutionary progress. These metrics should focus not just on current performance but on the system's capacity for continued growth and adaptation.
Through these structured approaches to architecture and implementation, organizations can build AI systems that don't just perform tasks but continuously evolve to meet changing business needs. The key is understanding that evolution in AI systems isn't a single feature or capability—it's a fundamental characteristic that shapes every aspect of system design and operation.
The next frontier in agentic AI isn't just about improving existing capabilities—it's about systems that can identify and develop entirely new competencies on their own. This represents a fundamental shift from our current approach to AI development.
Today's advanced systems can adapt within predefined parameters. Tomorrow's systems will redefine these parameters themselves. This isn't science fiction—we're already seeing early signs of this capability in experimental systems. These systems don't just optimize their performance; they actively identify new areas where they could provide value and develop the capabilities to do so.
Take the emerging field of autonomous problem identification. Future agentic systems won't wait for humans to point out issues or opportunities. They'll proactively scan their operational environment, identify potential challenges before they become problems, and develop solutions—all without direct human intervention.
Perhaps the most exciting development on the horizon is the emergence of meta-learning capabilities. These systems don't just learn from their experiences; they learn how to learn better. They continuously refine their learning processes, developing more efficient ways to acquire and apply new knowledge.
This meta-learning capability manifests in several groundbreaking ways:
The future of agentic AI lies not in isolated systems but in collaborative networks of specialized agents. These networks will operate much like human expert teams, with different agents bringing specialized knowledge and capabilities to solve complex problems.
This collaborative approach enables several powerful capabilities:
The emergence of collective intelligence that exceeds the sum of individual agents' capabilities. When multiple specialized agents work together, they create insights and solutions that none could achieve alone. This mirrors how human experts from different fields can combine their knowledge to solve previously intractable problems.
Dynamic team formation based on specific challenges. Rather than fixed configurations, these networks will automatically assemble the optimal combination of agents for each task. This flexibility ensures that every challenge is met with the most appropriate set of capabilities.
One of the most promising aspects of ecosystem evolution is the ability to transfer knowledge and capabilities across different domains. This isn't just about applying the same solutions to similar problems—it's about understanding the deep patterns that connect seemingly unrelated challenges.
This transfer manifests in fascinating ways:
As AI systems become more autonomous in their evolution, the need for effective governance becomes increasingly critical. This isn't about restricting development—it's about ensuring that evolution occurs in alignment with organisational goals and ethical principles.
Effective governance in evolving AI systems requires a new approach:
First, we need frameworks that can adapt as quickly as the systems they govern. Static rules and policies won't suffice when dealing with systems that continuously develop new capabilities. Instead, we need principles-based governance that can flex and scale with evolving systems.
Second, we must develop new ways to validate and verify evolutionary changes. Traditional testing approaches, designed for static systems, don't adequately address the challenges of systems that continuously evolve. We need new methodologies that can assess both immediate impacts and long-term implications of evolutionary changes.
As AI systems develop greater autonomy in their evolution, ensuring safety and ethical compliance becomes both more important and more challenging. This requires a sophisticated approach that goes beyond simple rule-based constraints.
Modern safety frameworks must address:
The evolution of agentic AI systems over the next five years will likely focus on several key areas:
Increased autonomy in capability development, with systems taking more initiative in identifying and pursuing new competencies. This will require sophisticated frameworks for ensuring these self-directed developments align with organizational goals.
More sophisticated collaboration between human experts and AI systems, moving beyond simple automation to true partnership in problem-solving and innovation.
The emergence of AI ecosystems where multiple evolving systems work together, creating networks of specialized capabilities that can tackle increasingly complex challenges.
This evolution represents not just a technical advancement but a fundamental shift in how we think about and work with artificial intelligence. Organizations that understand and embrace this shift will be best positioned to leverage these powerful new capabilities.
Fluid AI is an AI company based in Mumbai. We help organizations kickstart their AI journey. If you’re seeking a solution for your organization to enhance customer support, boost employee productivity and make the most of your organization’s data, look no further.
Take the first step on this exciting journey by booking a Free Discovery Call with us today and let us help you make your organization future-ready and unlock the full potential of AI for your organization.
AI-powered WhatsApp community for insights, support, and real-time collaboration.
Talk to our Enterprise GPT Specialists!
AI-powered WhatsApp community for insights, support, and real-time collaboration.