Get Fluid GPT for your organization and transform the way you work forever!
Talk to our GPT Specialist!
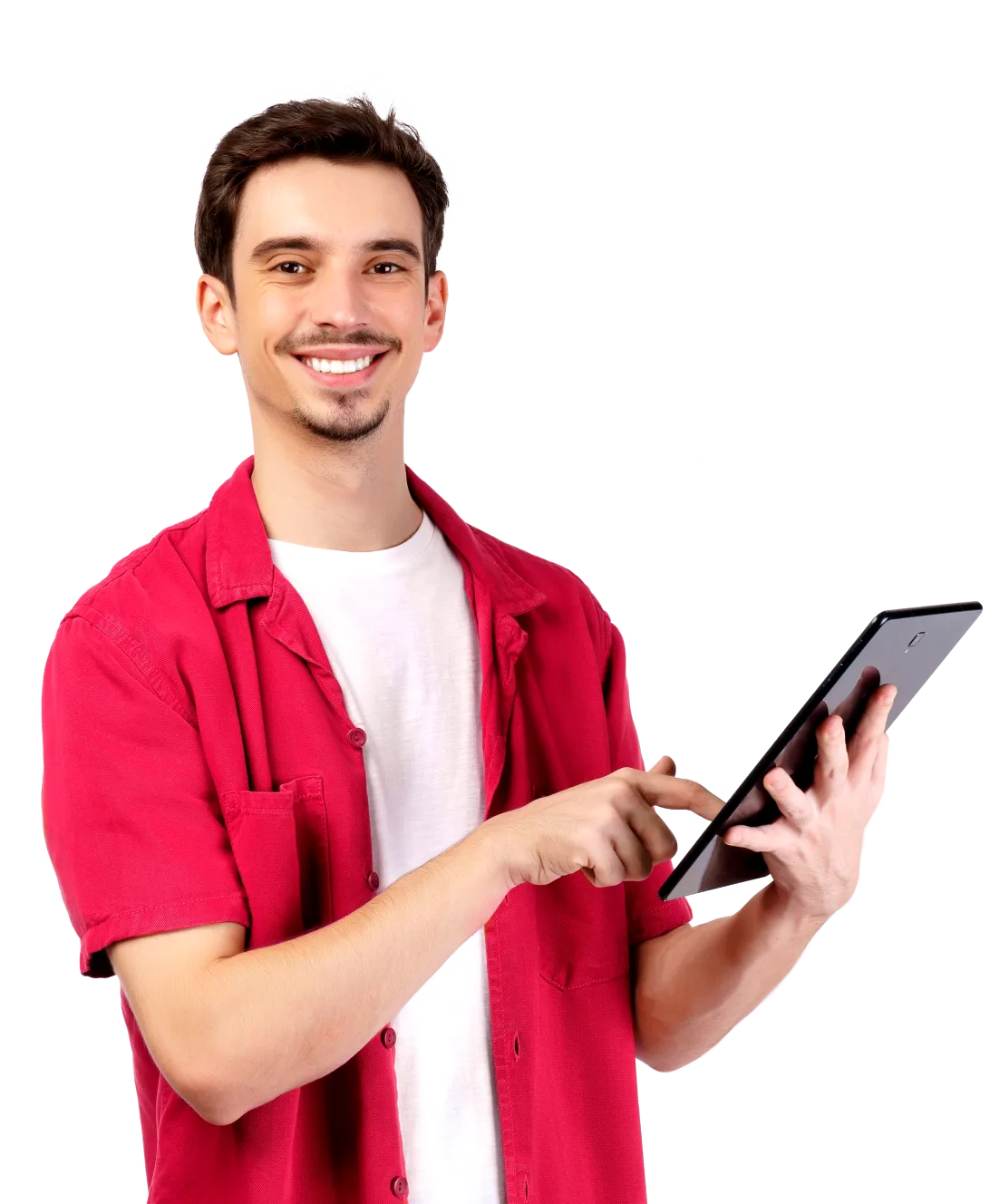
One of the main challenges in artificial intelligence (AI), especially in natural language processing (NLP), is to establish model correctness Conventional generative models are frequently unfounded in reality and suffer from genuine confusion. This can reduce the model's overall efficacy by producing outputs that are factually inaccurate yet grammatically correct.
Fortunately, a novel strategy called Retrieval Augmented Generation (RAG) is showing promise as a revolutionary idea. By incorporating retrieval techniques into the generation process, RAG makes it possible for models to obtain and utilize factual data from outside sources. This enables them to produce outputs that are more exact and honest, leading to a notable advancement in the dependability of AI.
Understanding Retrieval Augmented Generation
At its core, RAG operates in a two-stage process:
Benefits of Retrieval Augmented Generation
The integration of retrieval techniques into the generation process offers several advantages:
Real-World Applications of Retrieval Augmented Generation
The potential applications of RAG are vast and constantly evolving. Here are a few examples of how RAG is making waves in the AI landscape:
Conclusion: A Brighter Future for AI Accuracy
Retrieval Augmented Generation represents a significant advancement in the field of NLP. By integrating retrieval techniques into the generation process, RAG models pave the way for a future where AI outputs are not just grammatically correct but also factually grounded and reliable. This shift has the potential to revolutionize various AI applications, fostering trust and dependability in human-machine interactions. As RAG technology continues to evolve and knowledge bases become more comprehensive, we can expect even greater strides in AI accuracy and effectiveness.
Decision points | Open-Source LLM | Close-Source LLM |
---|---|---|
Accessibility | The code behind the LLM is freely available for anyone to inspect, modify, and use. This fosters collaboration and innovation. | The underlying code is proprietary and not accessible to the public. Users rely on the terms and conditions set by the developer. |
Customization | LLMs can be customized and adapted for specific tasks or applications. Developers can fine-tune the models and experiment with new techniques. | Customization options are typically limited. Users might have some options to adjust parameters, but are restricted to the functionalities provided by the developer. |
Community & Development | Benefit from a thriving community of developers and researchers who contribute to improvements, bug fixes, and feature enhancements. | Development is controlled by the owning company, with limited external contributions. |
Support | Support may come from the community, but users may need to rely on in-house expertise for troubleshooting and maintenance. | Typically comes with dedicated support from the developer, offering professional assistance and guidance. |
Cost | Generally free to use, with minimal costs for running the model on your own infrastructure, & may require investment in technical expertise for customization and maintenance. | May involve licensing fees, pay-per-use models or require cloud-based access with associated costs. |
Transparency & Bias | Greater transparency as the training data and methods are open to scrutiny, potentially reducing bias. | Limited transparency makes it harder to identify and address potential biases within the model. |
IP | Code and potentially training data are publicly accessible, can be used as a foundation for building new models. | Code and training data are considered trade secrets, no external contributions |
Security | Training data might be accessible, raising privacy concerns if it contains sensitive information & Security relies on the community | The codebase is not publicly accessible, control over the training data and stricter privacy measures & Security depends on the vendor's commitment |
Scalability | Users might need to invest in their own infrastructure to train and run very large models & require leveraging community experts resources | Companies often have access to significant resources for training and scaling their models and can be offered as cloud-based services |
Deployment & Integration Complexity | Offers greater flexibility for customization and integration into specific workflows but often requires more technical knowledge | Typically designed for ease of deployment and integration with minimal technical setup. Customization options might be limited to functionalities offered by the vendor. |
Talk to our GPT Specialist!