Tired of your data gathering dust ?
Lets put it to work with AI
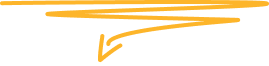
Talk to our Enterprise GPT Specialists!
This blog post aims to help organizations improve accuracy of their current RAG systems increasing trust and helping them become more flexible
Why is AI important in the banking sector? | The shift from traditional in-person banking to online and mobile platforms has increased customer demand for instant, personalized service. |
AI Virtual Assistants in Focus: | Banks are investing in AI-driven virtual assistants to create hyper-personalised, real-time solutions that improve customer experiences. |
What is the top challenge of using AI in banking? | Inefficiencies like higher Average Handling Time (AHT), lack of real-time data, and limited personalization hinder existing customer service strategies. |
Limits of Traditional Automation: | Automated systems need more nuanced queries, making them less effective for high-value customers with complex needs. |
What are the benefits of AI chatbots in Banking? | AI virtual assistants enhance efficiency, reduce operational costs, and empower CSRs by handling repetitive tasks and offering personalized interactions. |
Future Outlook of AI-enabled Virtual Assistants: | AI will transform the role of CSRs into more strategic, relationship-focused positions while continuing to elevate the customer experience in banking. |
Retrieval-Augmented Generation (RAG) is a technique that combines information retrieval with text generation, allowing AI models to retrieve relevant information from a knowledge source and incorporate it into generated text. RAG can enhance the accuracy and reliability of generative AI models, such as chatbots, by fetching facts from external sources, such as a knowledge base or a search engine.
However, RAG is not a silver bullet for all generative AI problems. Depending on the use case, RAG may have some limitations or challenges, such as:
Therefore, improving the accuracy of your RAG system requires careful attention to the following aspects:
The information retrieval system is responsible for finding and ranking the most relevant documents or passages from the knowledge source, given a user query. The information retrieval system can be based on different methods, such as keyword matching, vector similarity, or hybrid search.
Some of the techniques that can improve the information retrieval system are:
The knowledge source is the collection of documents or passages that provide grounding data for the generative AI models. The knowledge source can be based on different sources, such as Wikipedia, news articles, or domain-specific corpora.
Some of the techniques that can improve the knowledge source are:
The text generation system is responsible for producing natural and coherent text that answers the user query, given the retrieved information. The text generation system can be based on different models, such as LLAMA, GPT, or MISTRAL.
Some of the techniques that can improve the text generation system are:
Building an inhouse flexible and accurate RAG Systems is a little tricky. We at Fluid AI stand at the forefront of this AI revolution, helping organizations kickstart their AI journey helping them deploy a production ready RAG system within hours. We’re committed to making your organization future-ready, just like we’ve done for Mastercard, Bank of America, Warren Buffet and other top fortune 500 companies.
Take the first step towards this exciting journey by booking a free demo call with us today. Let’s explore the possibilities together and unlock the full potential of AI for your organization. Remember, the future belongs to those who prepare for it today.
AI-powered WhatsApp community for insights, support, and real-time collaboration.
Talk to our Enterprise GPT Specialists!
AI-powered WhatsApp community for insights, support, and real-time collaboration.