Start Your Transformation
with Fluid AI
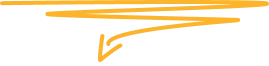
Join leading businesses using the
Agentic AI Platform to drive efficiency, innovation, and growth.
Explore how Agentic RAG is transforming AI with autonomous decision-making, real-time analysis, and adaptability across various industries like healthcare and finance.
Agentic RAG is reshaping how AI systems operate by incorporating autonomous agents that actively analyze and refine responses in real-time. Unlike Traditional RAG, which serves as a passive assistant by retrieving information upon request, Agentic RAG enables AI to act as a proactive partner, making real-time decisions independently. With applications across diverse fields such as healthcare, finance, and knowledge management, understanding the differences between these two types of RAG helps businesses choose the best AI solution for their needs.
Why is AI important in the banking sector? | The shift from traditional in-person banking to online and mobile platforms has increased customer demand for instant, personalized service. |
AI Virtual Assistants in Focus: | Banks are investing in AI-driven virtual assistants to create hyper-personalised, real-time solutions that improve customer experiences. |
What is the top challenge of using AI in banking? | Inefficiencies like higher Average Handling Time (AHT), lack of real-time data, and limited personalization hinder existing customer service strategies. |
Limits of Traditional Automation: | Automated systems need more nuanced queries, making them less effective for high-value customers with complex needs. |
What are the benefits of AI chatbots in Banking? | AI virtual assistants enhance efficiency, reduce operational costs, and empower CSRs by handling repetitive tasks and offering personalized interactions. |
Future Outlook of AI-enabled Virtual Assistants: | AI will transform the role of CSRs into more strategic, relationship-focused positions while continuing to elevate the customer experience in banking. |
AI is continuously advancing, from simple, rule-based systems to complex, data-driven decision-making. One of the major advancements in this evolution is Retrieval-Augmented Generation (RAG), an approach that combines data retrieval with natural language generation to provide context-rich responses across various industries. Whether in customer support, healthcare, or enterprise applications, RAG has opened doors to smarter and more responsive AI solutions.
If you're interested in exploring RAG technology more broadly, check out this overview on RAG Technology.
This blog will focus on the two main types of RAG systems:
Traditional RAG enhances AI’s capability to retrieve data and generate contextualized responses. It processes user inputs by pulling relevant data from various sources and then adds contextual information for a more tailored response. This form of RAG is commonly used in environments where accuracy in information retrieval is essential, such as customer support and FAQ systems.
Limitations: Traditional RAG works well for straightforward tasks but is limited in handling complex queries requiring iterative analysis or adaptive responses. For more insights on the practical applications and limitations of RAG systems, check out this in-depth guide on how organizations can improve their RAG systems.
Agentic RAG takes AI a step further by integrating intelligent agents capable of autonomous decision-making. Rather than simply retrieving information, Agentic RAG systems are designed to analyze data, refine responses iteratively, and adjust based on real-time feedback. This approach is powerful in complex settings, where dynamic data and multi-step reasoning are necessary.
Benefits of Agentic RAG:
Understanding the distinctions between Traditional and Agentic RAG systems can help businesses determine which solution best fits their needs:
Traditional RAG is efficient and effective for straightforward applications where response accuracy is a priority but complexity is minimal. Examples include:
In dynamic and high-stakes environments, Agentic RAG’s ability to autonomously refine responses is invaluable. Some key applications include:
For further exploration of Agentic AI’s role in the enterprise landscape, see this Agentic AI blog.
Traditional RAG is often preferred in settings where tasks are simple and predictable.
Agentic RAG, on the other hand, thrives in complex environments that require adaptability.
For insights into real-world uses of RAG, visit Beyond the Hype: Real-World Uses of RAG.
Agentic RAG plays a vital role in advancing autonomous AI systems that go beyond simple responses to proactively adapt based on real-time analysis. For instance, in predictive healthcare, Agentic RAG tracks and analyzes patient information to predict potential risks, offering proactive recommendations. This level of adaptability showcases the shift toward autonomous AI that operates independently, providing dynamic and self-sustaining solutions. For a deeper dive into the possibilities of Agentic AI, check out Exploring Autonomous AI Agents.
While Agentic RAG offers groundbreaking potential, ethical challenges must be addressed:
For further insights on responsible AI integration, read Fact-Checking Your AI.
Selecting the right RAG system depends on the business’s specific requirements:
Read more on how RAG improves AI accuracy.
The evolution from Traditional to Agentic RAG represents a groundbreaking shift in AI technology, transforming it from a passive assistant to a proactive, adaptive decision-maker. As businesses increasingly leverage AI to manage complex tasks, Agentic RAG provides a powerful tool for driving deeper insights, accelerating processes, and enabling autonomous responses. Embracing this technology can unlock new possibilities across healthcare, finance, and beyond, leading the way for the future of adaptive AI.
Fluid AI is an AI company based in Mumbai. We help organizations kickstart their AI journey. If you’re seeking a solution for your organization to enhance customer support, boost employee productivity and make the most of your organization’s data, look no further.
Take the first step on this exciting journey by booking a Free Discovery Call with us today and let us help you make your organization future-ready and unlock the full potential of AI for your organization.
AI-powered WhatsApp community for insights, support, and real-time collaboration.
Join leading businesses using the
Agentic AI Platform to drive efficiency, innovation, and growth.
AI-powered WhatsApp community for insights, support, and real-time collaboration.