Tired of your data gathering dust ?
Lets put it to work with AI
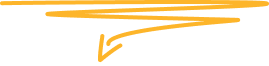
Talk to our Enterprise GPT Specialists!
Agentic RAG combines AI agents and retrieval systems for dynamic, precise decision-making, revolutionizing industries with smarter, adaptive workflows.
Agentic Retrieval-Augmented Generation (RAG) is transforming how businesses leverage AI for information retrieval and complex decision-making. By integrating intelligent agents into traditional RAG pipelines, Agentic RAG offers advanced reasoning, multi-step query handling, and tool adaptability.
This blog explores its core features, benefits, applications, and implementation strategies while highlighting real-world use cases and future trends. Discover how Agentic RAG is bridging the gap between traditional AI systems and next-generation enterprise solutions.
Why is AI important in the banking sector? | The shift from traditional in-person banking to online and mobile platforms has increased customer demand for instant, personalized service. |
AI Virtual Assistants in Focus: | Banks are investing in AI-driven virtual assistants to create hyper-personalised, real-time solutions that improve customer experiences. |
What is the top challenge of using AI in banking? | Inefficiencies like higher Average Handling Time (AHT), lack of real-time data, and limited personalization hinder existing customer service strategies. |
Limits of Traditional Automation: | Automated systems need more nuanced queries, making them less effective for high-value customers with complex needs. |
What are the benefits of AI chatbots in Banking? | AI virtual assistants enhance efficiency, reduce operational costs, and empower CSRs by handling repetitive tasks and offering personalized interactions. |
Future Outlook of AI-enabled Virtual Assistants: | AI will transform the role of CSRs into more strategic, relationship-focused positions while continuing to elevate the customer experience in banking. |
In the evolving AI landscape, Agentic Retrieval-Augmented Generation (RAG) is a game-changing advancement, bridging the gap between simple retrieval systems and sophisticated decision-making frameworks. By incorporating autonomous agents into RAG pipelines, businesses can now tackle intricate queries, analyze multi-faceted data, and make intelligent decisions with greater precision.
Unlike traditional RAG, which relies solely on linear retrieval, Agentic RAG integrates dynamic reasoning, enabling systems to understand the context and evaluate data quality to provide adaptive retrieval strategies for solutions. As enterprises push for faster, more accurate knowledge systems, Agentic RAG is emerging as the preferred solution across industries.
For a detailed comparison between Agentic RAG and traditional RAG, check out our comprehensive analysis here.
Agentic RAG takes the foundational Retrieval-Augmented Generation (RAG) model and supercharges it with AI agents that handle complex reasoning, thought chaining, and decision-making tasks. These agents act as intelligent orchestrators, managing components such as retrieval tools, query planning, and data synthesis.
Key features include:
For a deep dive into the foundational aspects of Agentic AI, explore our guide here.
To explore a deeper comparison and how these systems operate, read our in-depth blog: Agentic RAG vs. Traditional RAG: The Future of AI Decision-Making.
These simpler systems use one agent to handle tasks like retrieving and synthesizing data. They’re ideal for smaller-scale applications that require minimal orchestration.
In complex scenarios, multiple agents coordinate tasks, with each specializing in areas like retrieval, validation, or reasoning. These systems ensure scalability and precision by distributing workloads effectively.
Agentic RAG is playing a pivotal role in smart manufacturing, where dynamic workflows and real-time data are essential for operational success. By leveraging AI agents, manufacturers can optimize processes like inventory management, predictive maintenance, and robotic assembly. This allows businesses to streamline production while reducing costs and errors.
Discover how Agentic AI is revolutionizing manufacturing efficiency with actionable use cases in modern factories.
In the fast-paced world of telecommunications, Agentic RAG enhances customer support, network optimization, and service personalization. By integrating data from multiple channels, agents provide real-time insights that improve decision-making and customer satisfaction. This is transforming the telecom sector’s ability to handle complex workflows efficiently.
Agentic AI’s contributions to telecommunications advancements highlight its transformative potential in service delivery.
Organizations are integrating Agentic RAG to supercharge enterprise operations, automating workflows, improving data accessibility, and enabling smarter decision-making. These systems provide a seamless way to analyze data from diverse sources, helping businesses achieve operational excellence and scalability.
Explore how Agentic AI optimizes enterprise functions, ensuring agility and adaptability in competitive markets.
Selecting the right AI framework is essential for successfully implementing Agentic RAG. Platforms like LangChain and LlamaIndex offer modular architectures that simplify tool integration and task orchestration. These frameworks are particularly valuable for enterprises managing large-scale knowledge systems.
To maximize effectiveness, Agentic RAG systems rely on tailored knowledge bases. Incorporating domain-specific data ensures that retrieval and output processes align with organizational goals. Advanced systems can even integrate APIs and real-time databases to stay updated with changing requirements.
Continuous improvement is vital for Agentic RAG implementations. User feedback, paired with regular performance audits, helps refine retrieval strategies and ensures long-term success.
For businesses starting their journey, selecting the best AI agent requires understanding compatibility and scalability. Check out our guide to choosing the ideal AI agent for your needs for actionable insights.
As demand for more complex systems grows, multi-agent architectures will enable better task orchestration and collaborative decision-making.
Combining Agentic RAG with knowledge graphs will create even more powerful reasoning systems, capable of understanding intricate relationships between entities.
Future iterations of Agentic RAG will seamlessly process text, images, videos, and even IoT data, expanding its utility across industries.
Read about emerging trends in Agentic AI here.
Agentic RAG represents a monumental leap in AI-driven information retrieval, offering businesses unmatched capabilities in reasoning, adaptability, and decision-making. By integrating intelligent agents into RAG pipelines, enterprises can unlock new levels of efficiency and accuracy.
As industries continue to evolve, adopting Agentic RAG will no longer be optional but essential for staying competitive. Whether you’re building personalized assistants, streamlining enterprise workflows, or enhancing customer experiences, Agentic RAG is your gateway to the future of AI.
Fluid AI is an AI company based in Mumbai. We help organizations kickstart their AI journey. If you’re seeking a solution for your organization to enhance customer support, boost employee productivity and make the most of your organization’s data, look no further.
Take the first step on this exciting journey by booking a Free Discovery Call with us today and let us help you make your organization future-ready and unlock the full potential of AI for your organization.
AI-powered WhatsApp community for insights, support, and real-time collaboration.
Talk to our Enterprise GPT Specialists!
AI-powered WhatsApp community for insights, support, and real-time collaboration.